Choices you have when receiving data that has data quality issues
Found (via Twitter #DataQuality hashtag) a couple good postings on a blog called "Data and Process Advantage Blog" - here's some thoughts on the postings.
1) Are you a #DataQuality ostrich? Do you know an ostrich?
I enjoyed this entry and I do have a "small team assessing the completeness and validity of data using data profiling tools" but I'm hopeful we're not "avoiding doing data accuracy checking as it is seen as difficult".
2) An earlier posting named "Approaches to data quality issues from suppliers" really caught my attention - because in fact my shop does get data from many-many different data sources and each day we have to make very difficult decisions on what to do when we receive data with data quality issues. I may have posted this image before, but I'm a firm believer that you have four choices when receiving data with data quality issues:
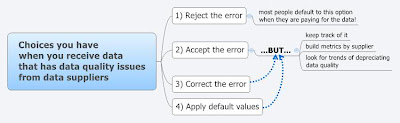
Just like everything else in life, one size solutions don't fit all.
In the blog entry, it sounds like the first organization followed the third approach. I'd guess however that they didn't follow through and keep track of changes as well as report those corrections back to their suppliers and to their clients or senior management. Had they done this they could have marketed the work they were doing as "value add" work as well as - well - just making the data better for the applications which use the data.
The second organization followed the path which I find most folks follow - they rejected the data. One major difference - they "did not state the nature of the errors" - very bold move here. Most folks go round after round with their data suppliers when rejecting data. Round one typically looks like "fields B and C are bad". Round two typically looks something like "how can field D have this value if field M has that value". Round three typically looks like "are we receiving all the data we're supposed to be getting?". Not telling the data supplier where the problems are feels weird to me. Imagine filling out an online form for ordering a product and when you hit submit it tells you "somethings wrong, go find the error Bozo"? I guess that would work if I was paying the person to fill out the form, I'm going to have to try this out some time.
If your pulling in data from data suppliers and you can't just plain reject the data, my thinking is that it's fair game to "add value" by correcting or applying default values. One note however - you MUST in fact keep track of the changes you've made so that everyone involved knows what was originally sent in and what data has "value added" from your team. Keeping track of such changes may or may not mitigate the risk of the "value add", I suppose this would have to be evaluated on a case-by-case basis.
As for choice three in my picture, I wish I could leave it out, but it is a valid choice. Just leave it alone and load it up into a database, again you should be able to easily identify what data actually has data issues.
If there are significant liability issues at stake for ensuring your data is accurate, you may not want to go down the path of correcting the data or you may want to try to add value. Either way, if you do in fact load it somewhere, please keep track of any changes you've made, you won't regret it.
I'm looking forward to more posts on the "Data and Process Advantage Blog".
Until next time...Rich
1) Are you a #DataQuality ostrich? Do you know an ostrich?
I enjoyed this entry and I do have a "small team assessing the completeness and validity of data using data profiling tools" but I'm hopeful we're not "avoiding doing data accuracy checking as it is seen as difficult".
2) An earlier posting named "Approaches to data quality issues from suppliers" really caught my attention - because in fact my shop does get data from many-many different data sources and each day we have to make very difficult decisions on what to do when we receive data with data quality issues. I may have posted this image before, but I'm a firm believer that you have four choices when receiving data with data quality issues:
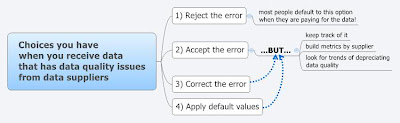
Just like everything else in life, one size solutions don't fit all.
In the blog entry, it sounds like the first organization followed the third approach. I'd guess however that they didn't follow through and keep track of changes as well as report those corrections back to their suppliers and to their clients or senior management. Had they done this they could have marketed the work they were doing as "value add" work as well as - well - just making the data better for the applications which use the data.
The second organization followed the path which I find most folks follow - they rejected the data. One major difference - they "did not state the nature of the errors" - very bold move here. Most folks go round after round with their data suppliers when rejecting data. Round one typically looks like "fields B and C are bad". Round two typically looks something like "how can field D have this value if field M has that value". Round three typically looks like "are we receiving all the data we're supposed to be getting?". Not telling the data supplier where the problems are feels weird to me. Imagine filling out an online form for ordering a product and when you hit submit it tells you "somethings wrong, go find the error Bozo"? I guess that would work if I was paying the person to fill out the form, I'm going to have to try this out some time.
If your pulling in data from data suppliers and you can't just plain reject the data, my thinking is that it's fair game to "add value" by correcting or applying default values. One note however - you MUST in fact keep track of the changes you've made so that everyone involved knows what was originally sent in and what data has "value added" from your team. Keeping track of such changes may or may not mitigate the risk of the "value add", I suppose this would have to be evaluated on a case-by-case basis.
As for choice three in my picture, I wish I could leave it out, but it is a valid choice. Just leave it alone and load it up into a database, again you should be able to easily identify what data actually has data issues.
If there are significant liability issues at stake for ensuring your data is accurate, you may not want to go down the path of correcting the data or you may want to try to add value. Either way, if you do in fact load it somewhere, please keep track of any changes you've made, you won't regret it.
I'm looking forward to more posts on the "Data and Process Advantage Blog".
Until next time...Rich
Comments
The suppliers in this case had a clear data spec. to comply with and are being paid to supply/change assets and supply the relevant data. The assets are safety critical and, if the data is wrong, could lead to substantial risks if people make decisions on incorrect data.
By rejecting the data without explanation, that forces the supplier to understand and comply with the standards. If you correct their errors for them, where is their incentive to supply good data?
- strengthened relationship with suppliers
- supplier process improvements
- improved trust in the data
- improved quality
Thanks!
I recently consulted with a company in the UK who had one guy working 3-4 days every month to cleanse a large data feed from a 3rd party. When I asked him why, the answers varied from "That's just how we do things" to "They're a much bigger company than us" so although it would seem perfectly logical to push back errors, logic doesn't always feature in DQ supplier management.
I think the approaches above are excellent as they demonstrate:
- Clear, agreed service levels/data spec.
- Financial incentive for compliance
- Working with the supplier to continuously improve
- Rigorous inbound data verification
- Full transparency, results are distributed immediately
Really great post, keep them coming!